286: [Sumedha Rai] The Future of AI and Your Wallet
- aboutthatwallet
- Feb 11
- 6 min read
Updated: Feb 24
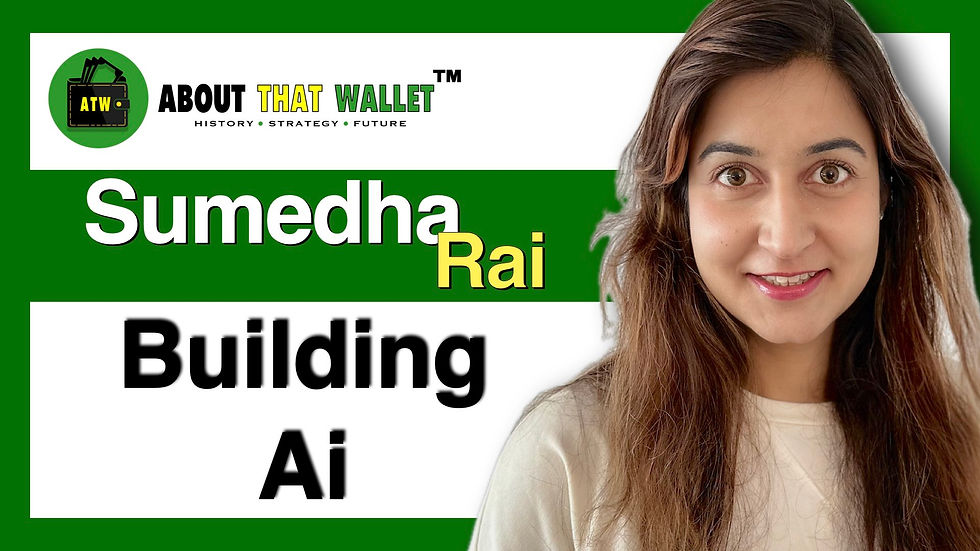
Table of contents:
Sumedha's website - https://sumedharai.podvantage.ai/
Poll:
How Should You Prepare for Ai?
Sacrifice personal savings to invest in Ai-focused education
Learn to use AI to automate Elder care
Refuse to adopt Ai solutions
Ignore Ai advancements and live your life
About Sumedha and the episode:
In the latest episode of our podcast, Anthony Weaver and Sumedha Rai dive deep into the fascinating intersection of artificial intelligence and financial literacy. The conversation centers around the growing importance of upskilling in a world increasingly dominated by technology. As AI continues to evolve, many individuals worry about job security and the potential for automation to replace human roles. However, our guest, Sumedha, emphasizes the importance of understanding AI and using it to enhance our skill sets rather than fearing it.
Sumedha shares her journey from a background in economics and finance to becoming an expert in AI, highlighting that anyone can transition into tech, regardless of their prior experience. She encourages listeners to engage with AI technologies by researching and understanding how they work, which will empower them to adapt to the changing job landscape.
One of the key takeaways from the episode is the necessity of financial literacy in today’s world. Sumedha discusses practical tools like budgeting apps and robo-advisors that can help individuals manage their finances more effectively. She stresses that letting money sit in a bank account without making it work for you is one of the worst mistakes people can make.
Moreover, the conversation touches on the ethical implications of AI, particularly regarding bias in decision-making processes. Sumedha urges companies to prioritize fairness and transparency in their AI systems to avoid perpetuating existing inequalities. She emphasizes the need for diverse data sets to create more equitable AI models, which ultimately leads to better outcomes for everyone.
Listeners are encouraged to take proactive steps in their financial journeys, whether that means investing in themselves through education or utilizing technology to enhance their financial decision-making. The episode serves as a reminder that knowledge is power, and in a rapidly changing world, staying informed and adaptable is crucial.
If you’re interested in learning how to navigate the complexities of AI and finance, this episode is a must-listen. Tune in to gain insights that could help you build a more secure financial future while embracing the advancements of technology.
Frequently Asked Questions (FAQ)
What does Sumedha Rai do as a data scientist at Acorns Grow?
As a data scientist at Acorns Grow, Sumedha Rai is responsible for creating end-to-end pipelines for processes that require data science models. This includes data ingestion, data wrangling, data mining, data cleaning, feature engineering, model building, and deployment. She works on a variety of projects, including unsupervised learning to understand customers and natural language processing (NLP) for both internal and external applications. She also works on supervised learning projects using models like XGBoost and Random Forest.
What programming skills and tools are most important for a data scientist, according to Sumedha Rai?
How did Sumedha Rai's non-traditional background in finance influence her data science career?
What strategies does Sumedha Rai recommend for identifying and mitigating bias in AI models, particularly in the context of fintech?
What are some ethical challenges facing AI development in finance, and how can they be addressed?
How can individuals with non-tech backgrounds break into the field of AI and data science?
How does Sumedha Rai define wealth and what advice does she give for personal finance management?
What role does interdisciplinary collaboration play in Sumedha Rai's approach to problem-solving?
Glossary of Key Terms
AI (Artificial Intelligence): The theory and development of computer systems able to perform tasks that normally require human intelligence, such as visual perception, speech recognition, decision-making, and translation between languages.
Bias (in AI): Systematic errors in AI model outputs due to flawed assumptions in the machine learning algorithm or biases in the training data.
Data Engineering: The aspect of data science that focuses on data collection and data ingestion.
Data Science: An interdisciplinary field that uses scientific methods, processes, algorithms, and systems to extract knowledge and insights from structured and unstructured data.
Feature Engineering: The process of selecting, transforming, and creating features from raw data to improve the performance of machine learning models.
Fintech: Financial technology; technology and innovation that aims to compete with traditional financial methods in the delivery of financial services.
Hallucinations (in AI): Instances where an AI model generates incorrect or nonsensical information that is not grounded in reality or the provided data.
LLM (Large Language Model): A type of AI model that is trained on a massive amount of text data and is capable of generating human-like text for a variety of tasks, such as language translation, text summarization, and question answering.
Machine Learning (ML): A type of artificial intelligence that enables computer systems to learn from data without being explicitly programmed.
Model Deployment: Making a machine learning model available for use in a production environment, enabling it to generate predictions on new data.
NLP (Natural Language Processing): A branch of AI that deals with the interaction between computers and humans using natural language.
Open Source Library: A collection of pre-written code, functions, or routines that is freely available for use and modification.
Productionizing: The process of deploying and scaling a machine learning model to be used in a real-world application or system.
Python: A high-level, general-purpose programming language widely used in data science and machine learning due to its versatility and extensive libraries.
Robo Advisor: An online platform that provides automated, algorithm-driven financial planning services with little to no human supervision.
SaaS (Software as a Service): A software distribution model in which a third-party provider hosts applications and makes them available to customers over the Internet.
Unsupervised Learning: A type of machine learning where the algorithm is trained on unlabeled data and aims to discover patterns, relationships, or structures within the data.
Comments